Could AI find alien life faster than humans, and would it tell us?
Machine-learning algorithms are transforming the search for extraterrestrial intelligence, finding candidate signals faster and better than ever before, but the development of artificial general intelligence could complicate contact.
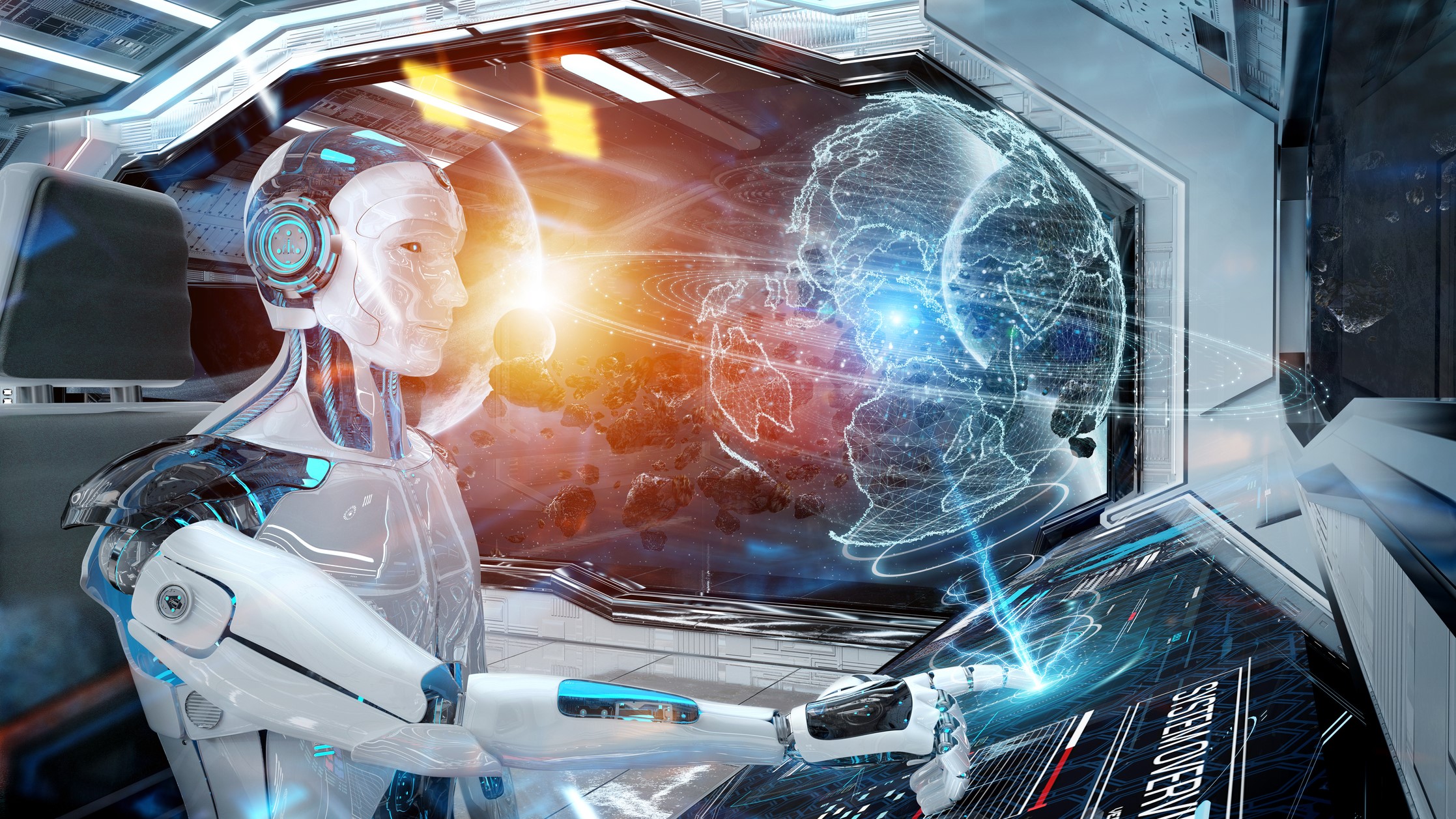
World Space Week 2023 is here and Space.com is looking at the current state of artificial intelligence (AI) and its impact on astronomy and space exploration as the space age celebrates its 66th anniversary. Here, Keith Cooper discusses how AI might help humans detect alien life.
Turn a radio telescope to the stars in the sky, and it's instantly deafened. From pulsars to radio galaxies, and ionospheric disturbances in the atmosphere to radio-frequency interference (RFI) from our own technology, the sky is a cacophony of radio noise. And somewhere, among all that, may lie a needle in a haystack: a signal from another world.
For over 60 years scientists have been scanning the skies in the search for extraterrestrial life but have yet to find any aliens. When you consider the sheer volume of search space — all those stars, all those radio frequencies — versus our limited searches so far, then it's little wonder we've not found ET yet. It's a daunting task, especially for a human.
Thankfully, we've got some non-human intelligence to join the search.
Related: AI is already helping astronomers make incredible discoveries. Here's how
The use of artificial intelligence (AI) is reaching critical mass, in our everyday lives and in science, so it is no surprise that it's now being employed in Search for Extraterrestrial Intelligence (SETI). We're not talking about Skynet, or the machines from The Matrix movies, or even Star Trek: The Next Generation's Data. The AI that is so in vogue at present is based on machine-learning algorithms designed to do very specific jobs, even if it's just to talk to you on ChatGPT.
To explain how AI is assisting in SETI, astronomer and SETI researcher Eamonn Kerins of the University of Manchester compares it to the needle in a haystack problem.
Get the Space.com Newsletter
Breaking space news, the latest updates on rocket launches, skywatching events and more!
"You basically treat the data as though it's the hay," Kerins told Space.com Space.com. "Then you're asking the machine-learning algorithm to tell you if there is anything in the data that isn't hay, and that hopefully is the needle in the haystack — unless there's other stuff in the haystack too."
That other stuff is usually RFI, but the machine-learning algorithm is trained to recognize all the types of RFI we already know about. Those signals — the familiar patterns of mobile phones, local radio transmitters, electronics and so on — are the hay.
The training involves "injecting signals into the data and then the algorithm learns to look for signals that are like that," Steve Croft, an astronomer with the Breakthrough Listen SETI project at the University of California, Berkeley, told Space.com The algorithm learns to spot the patterns of these familiar signals and disregard them. Should it spot something in the data that it hasn't been trained on, then it flags this up as something interesting that requires a human to follow up on.
"There have been attempts recently at sifting through some of the Breakthrough Listen data with a machine-learning algorithm," said Kerins. "The data had already been combed through quite carefully previously by more conventional means, but yet the algorithm was still able to pick out new signals after being trained on the stuff that we know about."
This project was led by Croft and an undergraduate student, Peter Ma of the University of Toronto, who wrote the algorithm and put it to work analyzing data from 820 stars observed by the 100-meter radio telescope at Green Bank Observatory in West Virginia. The data, totaling 489 hours' worth of observations, contained millions of radio signals, almost all of which were human-made interference. The algorithm checked every single one of them and found eight signals that did not match anything it had been trained on and which had been missed by earlier analyses of the data.
These eight signals seem to come from five different star systems, although they might be misleading. They haven't been detected since — to see a signal repeat is the most basic requirement for a signal to be considered interesting in SETI — and they will probably turn out to be more RFI. However, even that's useful, because they can be used to train the next generation of machine-learning AI so similar RFI can be avoided in the future.
Machine learning algorithms can be divided into two camps. One is known as supervised learning, which is the teach-it-everything-you-know approach. Unsupervised learning is a little different, in that you just feed the algorithm the data and let it figure out what is significant, without any human biases.
"With a fully unsupervised approach you just throw all the data in, stir the pot and let the algorithm figure it out by itself," said Croft.
As a mundane example, suppose you have a dataset of images of tables and chairs, and you want the algorithm to distinguish between them. In supervised learning, you train the algorithm on lots of images that are marked 'table' or 'chair'. With unsupervised learning, the algorithm has to distinguish between the two by grouping things that look similar without any prior training — for example, it might select anything with a back to be a chair, and anything with a long top to be a table.
Kerins highlights the example of a project led by Adam Lesnikowski of NVIDIA, who are famous for their graphics cards but which are now leaders in artificial intelligence. Lesnikowski, joined by Valentin Bickel of ETH Zurich and Daniel Angerhausen of the University of Bern, used unsupervised machine learning in a test to see whether it could spot artificial objects on the moon. The algorithm was fed images from NASA's Lunar Reconnaissance Orbiter, and it had to figure out what was a typical lunar feature, such as a crater or a rille, and what wasn't. The test was a success — the algorithm picked out the Apollo 15 lunar lander on the surface of the moon.
The idea is that technological aliens may have already visited our solar system, and left probes or artifacts on the planets, moons or asteroids. It's possible there may even be an active probe watching us right now.
"Some of my colleagues are very interested in the idea of having orbiters with a machine-learning algorithm on board," said Kerins. A spacecraft could survey planetary surfaces in our solar system to search for anomalies that could be alien probes, possibly millions or billions of years old now. Because unsupervised learning has the advantage of being able to function in real-time, it would be able to assess each image before moving on without having to wait to send all the data back to Earth for humans to look at.
Certainly, in the age of 'Big Data', machine-learning AI is the way forward and is now being used extensively in astronomy and in SETI, with the capability to do things faster and better than humans can.
"It's certainly fast," said Kerins. "The nearest we can get with humans is through citizen science projects."
With machine learning algorithms, humans are still intimately involved. A signal might get flagged up by the AI as being intriguing, but it is still humans who have to follow up and investigate. The algorithms aren't that smart.
A time may come soon, however, when they are that smart. Researchers at places such as Google DeepMind have been pursuing artificial general intelligence, or AGI. Whereas the algorithms we have today are very specific, AGI would be able to cast its hand to anything and learn and grow while it does. An AGI could rapidly accelerate beyond the capacity of human intelligence.
The possibilities for AGI transforming SETI are tantalizing. We've already seen how machine-learning algorithms designed to play games such as chess or Go! are developing strategies that befuddle the human experts whom the AI is beating in these games. An AGI could surely think of new ways in which to search for alien life beyond the confines of human biases and experience.
"It would be able to map out all sorts of possibilities for how language and communication can be conveyed through signals," said Kerins. "It might be able to consume vast astronomical catalogs and decide on optical strategies on how and where to look."
Steve Croft echoes Kerins' optimism. "I hope AI evolves to the stage where we can ask it to take the blinders off and imagine, from everything it knows about physics, biology, chemistry, exoplanets and technology, what it thinks ET might be doing. It will probably come up with some good ideas!"
That's if it can, or even will, tell us anything. The creation of an AGI will, in a way, by like creating an alien, one that is very much unlike us and which we might struggle to understand.
"We might find it very hard to directly communicate with it," said Kerins. "We might have some hierarchy of translators, and at the top of that hierarchy is an intelligence that would decide on much smarter ways to look in SETI. If it makes contact, then how does that filter down to the biological intelligences, the stupid guys, us?"
We might get a version of Chinese whispers, where the relevant information is passed down through the hierarchy, getting simpler and simpler until we receive the dumbed-down version. The AGI may even withhold information that it deems would be too complicated for us to understand. If AGI managed to make a SETI detection, we may not get the full picture.
That's speculation, though. In the here and now, AI is a powerful tool that is accelerating our searches for ET. It's a sure thing that if we do discover a signal from another world in the future, we'll have AI to thank for it.
Follow Keith Cooper on Twitter @21stCenturySETI.
Join our Space Forums to keep talking space on the latest missions, night sky and more! And if you have a news tip, correction or comment, let us know at: community@space.com.
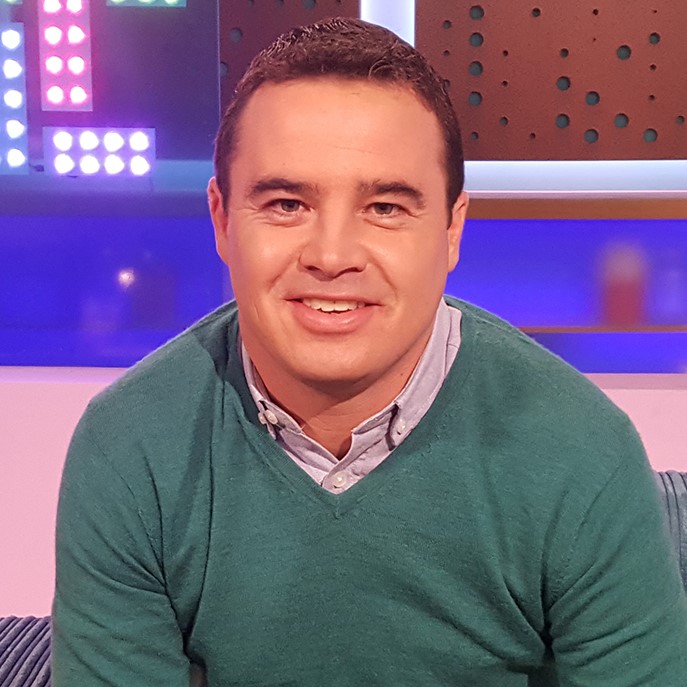
Keith Cooper is a freelance science journalist and editor in the United Kingdom, and has a degree in physics and astrophysics from the University of Manchester. He's the author of "The Contact Paradox: Challenging Our Assumptions in the Search for Extraterrestrial Intelligence" (Bloomsbury Sigma, 2020) and has written articles on astronomy, space, physics and astrobiology for a multitude of magazines and websites.
-
rod Perhaps this new approach to finding ET phoning home :) may have similar problems already discussed on the forums and articles.Reply
https://forums.space.com/threads/alien-life-may-not-be-carbon-based-new-study-suggests.63274/ -
bwana4swahili In all probability any alien 'life' that contacts us will be some form of AI so it makes sense for us to use AI to find it.Reply -
Jana Petreková In the end, a person will have to decide what is really intelligent. The analytical capacity of artificial intelligence is limited by the Margolus–Levitin theorem and Bremermann's limit. The human brain does not have these limitations because the concept of thought formation is the result of millions of years of complex evolutionary factors. Undoubtedly, alien intelligences will have a similar genesis of brain development, and their artificial intelligences will have the same limitations as human artificial intelligences.Reply -
Dave In the future it is possible that the human mind may have direct access (integrated) with both computer and AI technologies. Imagine what the next Einstein could achieve.Reply