The 1st-ever photo of a supermassive black hole just got an AI 'makeover' and it looks absolutely amazing
The image of the supermassive black hole at the heart of the galaxy Messier 87 was boosted to high fidelity by a machine learning program trained on black hole models.
A distant supermassive black hole is looking sharp after a makeover from a supercomputer.
The "fuzzy orange donut" seen in the first image of a black hole ever taken has slimmed down to a thinner "skinny golden ring" with the aid of machine learning.
The redefinition of this image of the supermassive black hole at the heart of the galaxy Messier 87 (M87) could help better understand its characteristics and could be extended to the black hole at the heart of our own galaxy, the Milky Way.
The historic image of the M87 supermassive black hole, known as M87*, was taken by the Event Horizon Telescope (EHT) and was revealed to the public in 2019. The data to create the image was collected by the EHT over several days in 2017.
Related: First image of a black hole gets a polarizing update that sheds light on magnetic fields
The EHT is a network of seven telescopes across the globe that creates an Earth-sized telescope, but despite its combined observing power, there are still gaps in the data it collects, much like the missing pieces of a jigsaw puzzle.
A team of researchers including EHT collaboration member and astrophysics postdoctoral fellow Lia Medeiros, used a new machine learning technique called principal-component interferometric modeling or "PRIMO" to "fill in the gaps" in the M87 image and boost the EHT array to its maximum resolution for the first time.
Get the Space.com Newsletter
Breaking space news, the latest updates on rocket launches, skywatching events and more!
"Since we cannot study black holes up close, the detail of an image plays a critical role in our ability to understand its behavior," research lead author Medeiros said in a statement. "The width of the ring in the image is now smaller by about a factor of two, which will be a powerful constraint for our theoretical models and tests of gravity."
When the image of the M87 supermassive black hole (M87*), which is 55 million light-years from Earth and has a mass equivalent to six and a half billion suns, was first revealed, scientists were astounded about just how well it matched predictions made by Albert Einstein's 1915 general theory of relativity.
This PRIMO refined image of M87* gives scientists a chance to better match observations of an actual black hole to theoretical predictions.
"PRIMO is a new approach to the difficult task of constructing images from EHT observations," EHT member and NOIRLab researcher Tod Lauer said in the statement. "It provides a way to compensate for the missing information about the object being observed, which is required to generate the image that would have been seen using a single gigantic radio telescope the size of the Earth."
Training PRIMO to build a better black hole
The Institute for Advanced Study in Princeton, New Jersey explained that PRIMO operates using dictionary learning, a branch of machine learning which enables computers to generate rules based on large sets of training material. So for example, if a program like this is fed a number of images of a banana it can learn to determine if an image of an unknown object is a banana or not.
To train PRIMO to do the same thing with black holes the team fed it 30,000 high-fidelity simulated images of these cosmic titans as they feed on surrounding gas, a process called "accretion." The images covered a wide spread of theoretical predictions of how black holes accrete matter allowing PRIMO to hunt for patterns.
Once identified, these patterns were sorted based on how often they factored into simulations. This could then be incorporated into EHT images to create a high-fidelity image of M87* and reveal structures the telescope array may have missed.
"We are using physics to fill in regions of missing data in a way that has never been done before by using machine learning," Medeiros explained. "This could have important implications for interferometry, which plays a role in fields from exoplanets to medicine."
The resultant image rendered by PRIMO agrees with EHT data and theoretical black hole models. These models explain that the bright ring seen in these images of M87* is the result of gas being accelerated to near-light speeds by the incredible gravitational influence of the black hole. This causes the gas to heat up and glow as it whips around the light-trapping surface that forms the outer bounds of the black hole called the event horizon.
"Approximately four years after the first horizon-scale image of a black hole was unveiled by EHT in 2019, we have marked another milestone, producing an image that utilizes the full resolution of the array for the first time," stated Psaltis. "The new machine learning techniques that we have developed provide a golden opportunity for our collective work to understand black hole physics."
The PRIMO technique could now be applied to the image of the supermassive black hole at the heart of our galaxy, the Milky Way. The EHT revealed an image of this smaller but much closer supermassive black hole called Sagittarius A* (Sgr A*) in May 2022. The image of Sgr A* was created using data from the EHT also collected in 2017, but the smaller size of this four million solar mass black hole located 26,000 light-years from Earth in comparison to M87* made the data more difficult to refine.
Using PRIMO to boost the resolution of EHT images could help better refine estimations of the characteristics of both supermassive black holes including their mass, size, and the rate at which they consume matter.
"The 2019 image was just the beginning," Medeiros concluded. "If a picture is worth a thousand words, the data underlying that image have many more stories to tell. PRIMO will continue to be a critical tool in extracting such insights."
The team's research was published on April 13 in the Astrophysical Journal Letters.
Follow us on Twitter @Spacedotcom or on Facebook.
Join our Space Forums to keep talking space on the latest missions, night sky and more! And if you have a news tip, correction or comment, let us know at: community@space.com.
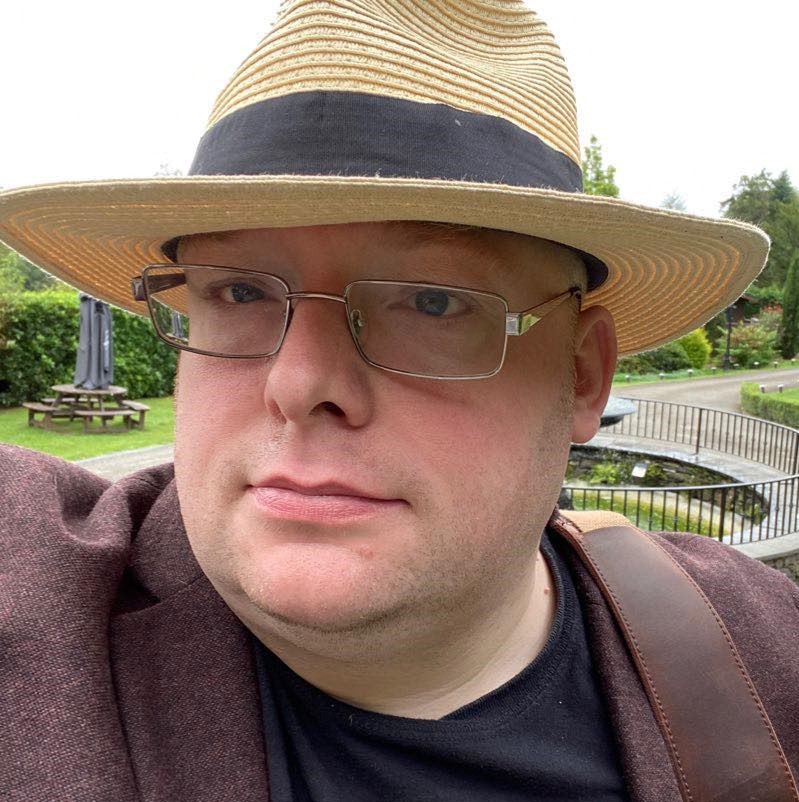
Robert Lea is a science journalist in the U.K. whose articles have been published in Physics World, New Scientist, Astronomy Magazine, All About Space, Newsweek and ZME Science. He also writes about science communication for Elsevier and the European Journal of Physics. Rob holds a bachelor of science degree in physics and astronomy from the U.K.’s Open University. Follow him on Twitter @sciencef1rst.
-
Sparky64 So the AI changed some settings to the image and wha-la it is sharper! Are you saying a human could not have made the same adjustments to the image? I mean how in the world can something still basically in it's infancy know what a black hole really looks like? It doesn't and anyone could have made those image adjustments to sharpen the image.Reply
Now don't get me wrong, I think there is a great future ahead for AI and all that it can do for the betterment of mankind. But this is like proud parents talking about how wonderful their baby child put a round peg in a round hole while drooling all over the place. Trained monkeys can do the same thing. This is not news worthy by any stretch. Proud parent moment yes, but not news worthy. -
billslugg The second image bears little resemblance to the first.Reply
Without knowning what rules were applied, the aec=xercise has no value.
Has it been replicated on a different AI machine?
Just ignore it.