Computer Program Learns to Sort Galaxies Like a Human
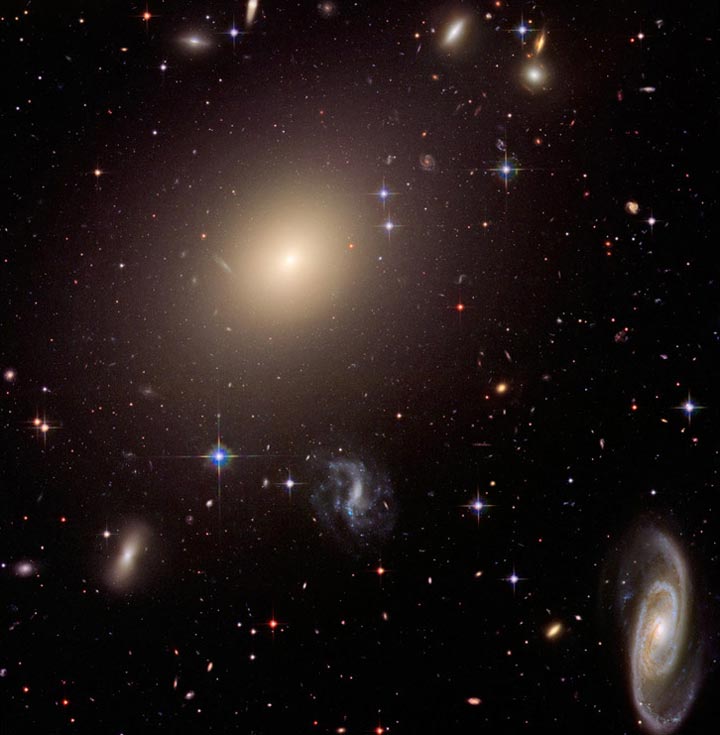
A computer algorithm modeled after the human brain haslearned how to recognize different galaxy types ranging from spiral toelliptical, and can now help flesh-and-blood stargazers with the daunting taskof classifying billions of galaxies.
The machine-learning codes have proven reliable enough toagree with human classifications of galaxies90 percent of the time, according to scientists at University College Londonand the University of Cambridge in the UK.
That should help astronomers keep up with a deluge of galaxyimagery from observational projects such as the Sloan Digital Sky Survey andthe Galaxy Zoo. The billions of galaxies in the knownuniverse include a widerange of shapes such as spiral, elliptical, barred and irregular.
"Next generation telescopes now under construction willimage hundreds of millions and even billions of galaxies over the comingdecade," said Manda Banerji, an astronomer at the University of Cambridge."The numbers are overwhelming and every image cannot viably be studied bythe human eye."
Classifying the types of galaxies represents the first steptoward understanding the origin and evolution of galaxies.
More than 250,000 people have helped astronomers classify 60million galaxies in the online Galaxy Zoo project. Astronomers then used theGalaxy Zoo classifications to train their computer algorithm, known as anartificial neural network, as part of the process for learning to recognizegalaxy types.
The artificial neural network can analyze the complexrelationships between different variables such as shape, size and color ofastrophysical objects, and then come up with the appropriate galaxy type. Thatprocess mimics the biological neural network found in living creatures.
Breaking space news, the latest updates on rocket launches, skywatching events and more!
Astronomers first trained the computer algorithm on 75,000astrophysical objects from the Sloan Digital Sky Survey included in the GalaxyZoo project, before testing its abilities in classifying 1 million objects.They also fiddled with the weighted parameters that the algorithm used so thatthey could finally achieve the 90 percent success rate.
Some limitations exist. For instance, the algorithm had aproblem with misclassifying red spirals and blue ellipticals, because too fewexamples of those objects exist for the algorithm to get a good read.
The study, published in the journal Monthly Notices ofthe Royal Astronomical Society, also did not include intermediary objectssuch as galaxymergers that defy easy classification.
Still, even a limited auto-classification method shouldprove useful for astronomers drowning in galaxy imagery and data. One recentcelestial census even found that astronomers had been undercounting the numberof distant galaxies by about 90 percent.
"While human eyes are very efficient in recognizingpatterns, clever computational techniques that can reproduce this behavior areessential as we begin to push the boundaries of our observable Universe anddetect more distant galaxies," said Ofer Lahav, an astrophysicist at theUniversity College London. "This study is an important step in thatdirection."
- Gallery - When Galaxies Collide
- The Strangest Things in Space
- Galaxies Undercounted in Cosmic Census
Join our Space Forums to keep talking space on the latest missions, night sky and more! And if you have a news tip, correction or comment, let us know at: community@space.com.
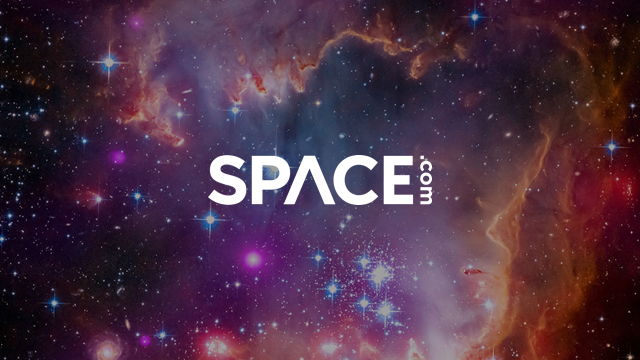
Space.com is the premier source of space exploration, innovation and astronomy news, chronicling (and celebrating) humanity's ongoing expansion across the final frontier. Originally founded in 1999, Space.com is, and always has been, the passion of writers and editors who are space fans and also trained journalists. Our current news team consists of Editor-in-Chief Tariq Malik; Editor Hanneke Weitering, Senior Space Writer Mike Wall; Senior Writer Meghan Bartels; Senior Writer Chelsea Gohd, Senior Writer Tereza Pultarova and Staff Writer Alexander Cox, focusing on e-commerce. Senior Producer Steve Spaleta oversees our space videos, with Diana Whitcroft as our Social Media Editor.